Research Groups
The Max Planck Institute for Intelligent Systems currently houses multiple Research Groups, all of which are lead by outstanding scientists who receive a secure budget. This freedom helps them propel their research and lay the foundations for a successful scientific career.
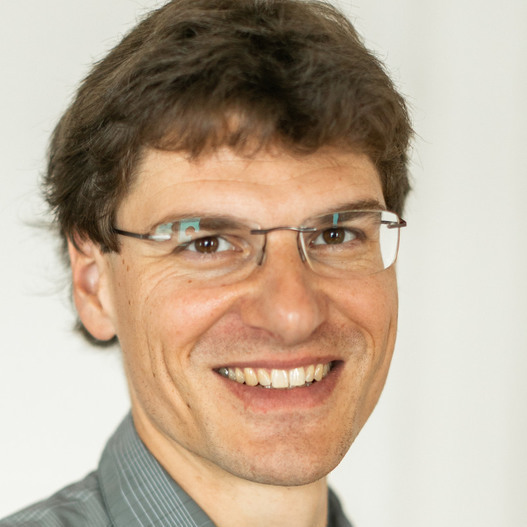
Autonomous Learning
Georg Martius
The Autonomous Learning group’s mission is to make robots learn in a way that is similar to early childhood development. Learning directly from interacting with their environment can potentially enable them to act in a complex and constantly changing world, which they cannot be programmed to do.
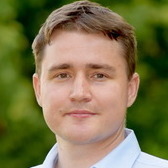
Dynamic Locomotion
Alexander Badri-Sprowitz
Animals not only run dynamically, efficiently, and elegantly, they also quickly adapt to new terrain while moving on it. Their locomotion is a carefully orchestrated interplay of muscles and tendons that has been optimized over the course of evolution. Alexander Badri-Spröwitz and his team use robots and simulations to understand animals and their movements. They investigate why an animal activates a muscle, what forces enable the animal to move or why not all muscles and tendons are the same. The researchers take inspiration from animals to build robot models. With robots, researchers can directly test the function of the individual parts. Their findings could help to improve walking robots, prostheses or exoskeleton technologies – external support structures for the body.
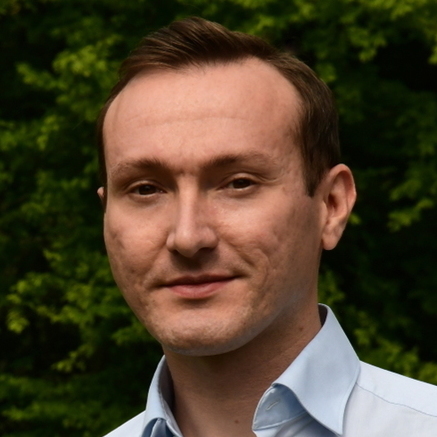
Embodied Vision
Jörg Stückler
Intelligent systems such as robots need the ability to learn and adapt to their environment. The Embodied Vision research group investigates novel methods that make it possible to understand dynamic 3D scenes. Such knowledge is required for artificial intelligent systems to solve complex tasks such as autonomous navigation or object manipulation.
Most common approaches that enable robots to carry out specific tasks are based on different components for perception and control. In contrast, the group’s researchers develop holistic methods that allow robots to learn how to interact with the environment and perform tasks. To do this, the robots should learn to draw on the sensor data of cameras and touch sensors to develop a model of their surroundings that is related to their task. They should also learn to predict the immediate effects of their actions. In turn, the knowledge they acquire from the model could enable robots to choose their actions themselves.
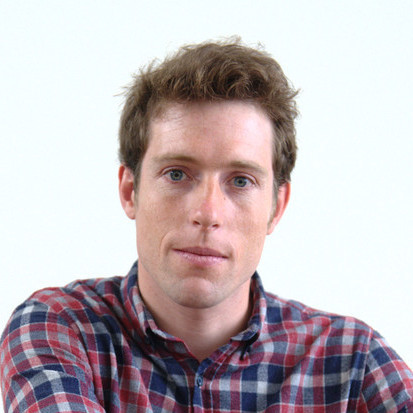
Learning and Dynamical Systems
Michael Mühlebach
Machine learning algorithms, which aim at extracting and recognizing patterns from observed data, will play a central role for enabling robotic systems that efficiently and seamlessly adapt to changing environments. While current supervised learning techniques have been very successful at tasks such as image recognition, speech recognition, or personalized recommendations, their extension to cyber-physical and robotic systems leads to many challenges. A promising approach to deal with these challenges is to incorporate the wealth of a-priori known structure that many robotic and cyber-physical systems have, such as approximate models based on first principles, symmetries, and invariants. This could improve the sample complexity, ensure that the predictions generalize to unseen situations, and could also facilitate down-stream tasks.
At the Learning and Dynamical Systems Group we seek to extend techniques from machine learning, dynamical systems, and control theory for enabling future cyber-physical and robotic systems. While rigorous theory and mathematical analysis forms the basis of our research, we also evaluate our methods in experiments on real-world systems.
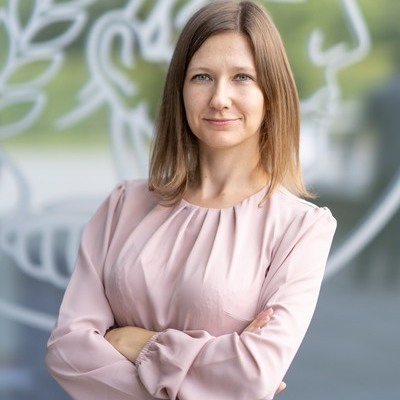
Organizational Leadership and Diversity
Ksenia Keplinger
The vision of the “Organizational Leadership and Diversity” group is to support leaders in using artificial intelligence to contribute to more tolerant, diverse, and inclusive organizations. We use qualitative (interviews, observations), quantitative (online experiments, lab experiments, field surveys), and mixed-methods to 1) investigate the use of artificial intelligence tools in the workplace to unleash the true potential of diversity and inclusion, 2) develop ways to mitigate bias in human-machine partnership, and 3) explore the nature of leadership in the artificial intelligence age.
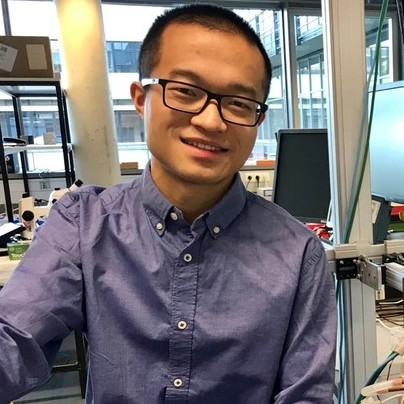
Bioinspired Autonomous Miniature Robots
Wenqi Hu
Our group focuses on resolving the scientific challenge of achieving unprecedented robotic performance by (Area-1) developing smart robotic materials and then (Area-2) integrating them into complex functional robotic systems.
In Area-1, we’d like to develop materials to meet the requirement of robotics. This includes better controllability (behavior is deterministic and predictable) and reliability. At the next level, we want to make materials with embedded intelligence that can realize these properties more elegantly.
In Area-2, we have to point out that the integration is a grand challenge even though there are existing materials that are good in each specialized function. Therefore, it is very challenging to integrate them together due to conflicting requirements and the demand for compatible interfaces. To address the challenge of integration, we are developing different integration strategies and their corresponding fabrication methods.
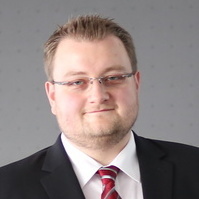
Neural Capture & Synthesis
Justus Thies
The Neural Capture and Synthesis Group works at the intersection of computer graphics, computer vision and machine learning. Specifically, I am interested in marker-less motion capturing of facial performances, human bodies as well as general non-rigid objects. Besides capturing and reconstructing reality, I work on AI-based synthesis techniques that allow for photorealistic image and video synthesis.
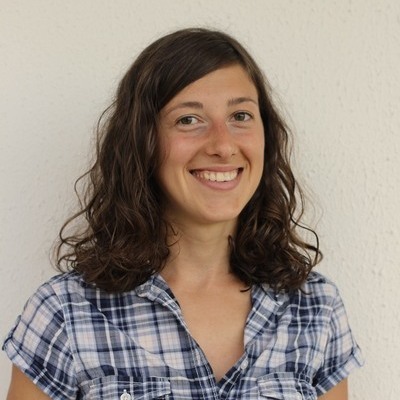
Physics for Inference and Optimization
Caterina De Bacco
The Physics for Inference and Optimization Group’s research focuses on understanding relations between the microscopic and macroscopic properties of complex large-scale interacting systems, such as networks. In cooperation with experts from other disciplines, De Bacco and her team develop models and algorithms based on principles of statistical physics. This knowledge could be used, for instance, to modify the interactions of a network’s individual constituents and thus to optimize its overall properties.
One of the group’s research interests is routing optimization. By collecting data on individual drivers and the vehicles in their immediate surroundings, researchers can draw conclusions on the behavioral patterns of this small group, and use these to make a generalization about all driving behaviors. In other words, they zoom in on part of the whole, closely observe behavioral patterns, and project their findings onto the big picture. Such knowledge can be used to calculate the best possible individual routes for all drivers by optimizing traffic management, even if this may mean a longer distance for the individual. In a collaboration with the Mathematics Department at the University of Padova, De Bacco’s group developed an efficient algorithm capable of deriving optimal general solutions for many routing problems.
De Bacco and her team also focus on investigating inference problems on networks. Inference aims to estimate the parameters of a model that is believed to have generated certain data. De Bacco investigates, for example, how likely it is that members of a social network will interact with one another. In this research area, for instance, she and her group recently developed a model that serves to estimate a node’s measure of importance in a network (known as eigenvector centrality) from a graph sample. This measure is particularly relevant when retrieving the information for the whole network is not feasible, as is the case with social networks.
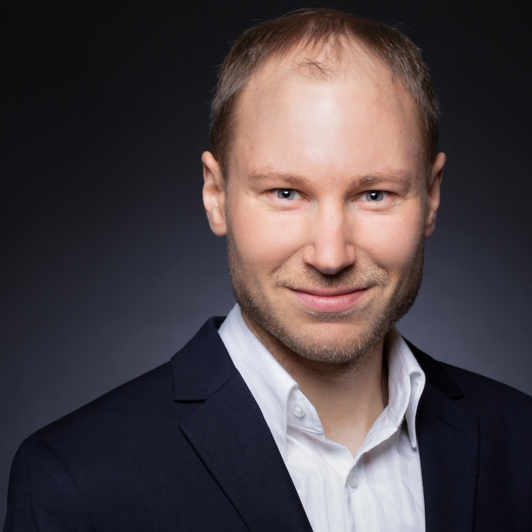
Rationality Enhancement
Falk Lieder
The Max Planck Research Group for Rationality Enhancement aims to develop a scientific foundation and intelligent technologies that enable people to become more effective. To this end, its research focuses on cognitive growth, goal setting, and goal achievement. The group elucidates the underlying mechanisms and investigates how they can be promoted, supported, and improved. If it succeeds, the group’s findings will revolutionize self-improvement, personal development, psychiatry and psychotherapy, brain training, and education.
Here you can additionally find information about Archived Departments and Research Groups (independent research group) that belonged to our institute for several years. Usually there is still a close scientific exchange between them and us, up to cooperation in scientific projects. The presence on our website shows the research activities of the groups during their time at MPI-IS.